
BOOKS - Data Analytics and Machine Learning for Integrated Corridor Management

Data Analytics and Machine Learning for Integrated Corridor Management
Author: Yashaswi Karnati, Dhruv Mahajan, Tania Banerjee, Rahul Sengupta, Clay Packard, Ryan Casburn
Year: 2025
Format: PDF | EPUB
File size: 33.4 MB
Language: ENG
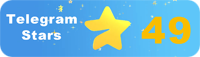
Year: 2025
Format: PDF | EPUB
File size: 33.4 MB
Language: ENG
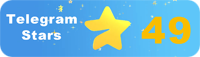
The book "Data Analytics and Machine Learning for Integrated Corridor Management" explores the potential of data analytics and machine learning in integrated corridor management, highlighting the importance of these technologies in optimizing transportation infrastructure planning, design, operation, and maintenance. The book provides insights into how data analytics and machine learning can be applied to various transportation modes, including roads, railways, airports, seaports, and public transportation systems. It covers topics such as data-driven decision-making, predictive modeling, and intelligent transportation systems, and offers practical examples of successful applications of these technologies in different regions of the world. The book begins by discussing the evolution of technology and its impact on society, emphasizing the need for a personal paradigm shift in understanding the technological process of developing modern knowledge. This shift involves recognizing the interconnectedness of technology and society and the imperative of adapting to new technologies to ensure human survival and the unification of people in a warring state. The author argues that this shift is necessary to overcome the challenges posed by the rapid pace of technological change and the increasing complexity of modern life. The book then delves into the specifics of data analytics and machine learning, explaining their potential to transform integrated corridor management. It explores the use of data analytics in traffic flow prediction, route optimization, and traveler information systems, as well as the application of machine learning algorithms to improve traffic management and reduce congestion. The author highlights the importance of integrating data analytics and machine learning into existing transportation infrastructure and operations, emphasizing the need for a comprehensive approach to managing corridors that considers all modes of transportation.
Книга «Data Analytics and Machine arning for Integrated Corridor Management» исследует потенциал аналитики данных и машинного обучения в интегрированном управлении коридорами, подчеркивая важность этих технологий в оптимизации планирования, проектирования, эксплуатации и обслуживания транспортной инфраструктуры. Книга дает представление о том, как аналитика данных и машинное обучение могут быть применены к различным видам транспорта, включая автомобильные дороги, железные дороги, аэропорты, морские порты и системы общественного транспорта. Он охватывает такие темы, как принятие решений на основе данных, прогнозное моделирование и интеллектуальные транспортные системы, и предлагает практические примеры успешного применения этих технологий в разных регионах мира. Книга начинается с обсуждения эволюции технологий и их влияния на общество, подчёркивая необходимость личностной смены парадигмы в понимании технологического процесса развития современных знаний. Этот сдвиг предполагает признание взаимосвязанности технологий и общества и императива адаптации к новым технологиям для обеспечения выживания человека и объединения людей в воюющем государстве. Автор утверждает, что этот сдвиг необходим для преодоления проблем, связанных с быстрыми темпами технологических изменений и возрастающей сложностью современной жизни. Затем книга углубляется в особенности аналитики данных и машинного обучения, объясняя их потенциал для трансформации интегрированного управления коридорами. В нем исследуется использование аналитики данных в прогнозировании потоков трафика, оптимизации маршрутов и информационных системах путешественников, а также применение алгоритмов машинного обучения для улучшения управления трафиком и уменьшения заторов. Автор подчеркивает важность интеграции аналитики данных и машинного обучения в существующую транспортную инфраструктуру и операции, подчеркивая необходимость комплексного подхода к управлению коридорами, учитывающего все виды транспорта.
''
