
BOOKS - Prompt Engineering for Generative AI: Future-Proof Inputs for Reliable AI Out...

Prompt Engineering for Generative AI: Future-Proof Inputs for Reliable AI Outputs
Author: James Phoenix
Year: June 25, 2024
Format: PDF
File size: PDF 45 MB
Language: English
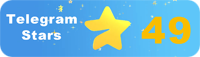
Year: June 25, 2024
Format: PDF
File size: PDF 45 MB
Language: English
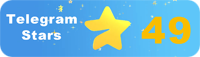
Large language models (LLMs) and diffusion models like ChatGPT and Stable Diffusion have shown great potential because they have been trained on all public text and images online, making them useful for a wide range of tasks. These models have the potential to revolutionize various industries, from healthcare to finance to education. However, integrating these models into automated systems poses significant challenges, such as obtaining reliable results.
In their book "Prompt Engineering for Generative AI FutureProof Inputs for Reliable AI Outputs James Phoenix and Mike Taylor demonstrate how to use a set of principles called prompt engineering to effectively work with AI. The authors show that by understanding the process of technology evolution, developing a personal paradigm for perceiving the technological process of modern knowledge development, and studying the survival of humanity and unity in a warring state, we can harness the power of LLMs and diffusion models to tackle problems previously unsuitable for automation.
The book begins by exploring the need to study and comprehend the process of technological advancement. The authors argue that understanding the evolution of technology is essential for harnessing its full potential.
In their book "Prompt Engineering for Generative AI FutureProof Inputs for Reliable AI Outputs James Phoenix and Mike Taylor demonstrate how to use a set of principles called prompt engineering to effectively work with AI. The authors show that by understanding the process of technology evolution, developing a personal paradigm for perceiving the technological process of modern knowledge development, and studying the survival of humanity and unity in a warring state, we can harness the power of LLMs and diffusion models to tackle problems previously unsuitable for automation.
The book begins by exploring the need to study and comprehend the process of technological advancement. The authors argue that understanding the evolution of technology is essential for harnessing its full potential.
Модели больших языков (LLM) и диффузионные модели, такие как ChatGPT и Stable Diffusion, показали большой потенциал, потому что они были обучены всему публичному тексту и изображениям в Интернете, что делает их полезными для широкого спектра задач. Эти модели могут революционизировать различные отрасли, от здравоохранения до финансов и образования. Однако интеграция этих моделей в автоматизированные системы создает значительные проблемы, такие как получение надежных результатов.
В своей книге «Prompt Engineering for Generative AI FutureProof Inputs for Reliable AI Outputs» Джеймс Феникс (James Phoenix) и Майк Тейлор (Mike Taylor) демонстрируют, как использовать набор принципов, называемых prompt engineering, для эффективной работы с ИИ. Авторы показывают, что, понимая процесс эволюции технологий, разрабатывая персональную парадигму восприятия технологического процесса развития современных знаний и изучая выживание человечества и единство в воюющем государстве, мы можем использовать мощь LLM и диффузионных моделей для решения проблем, ранее непригодных для автоматизации.
Книга начинается с изучения необходимости изучения и понимания процесса технологического прогресса. Авторы утверждают, что понимание эволюции технологий необходимо для полного использования их потенциала.
В своей книге «Prompt Engineering for Generative AI FutureProof Inputs for Reliable AI Outputs» Джеймс Феникс (James Phoenix) и Майк Тейлор (Mike Taylor) демонстрируют, как использовать набор принципов, называемых prompt engineering, для эффективной работы с ИИ. Авторы показывают, что, понимая процесс эволюции технологий, разрабатывая персональную парадигму восприятия технологического процесса развития современных знаний и изучая выживание человечества и единство в воюющем государстве, мы можем использовать мощь LLM и диффузионных моделей для решения проблем, ранее непригодных для автоматизации.
Книга начинается с изучения необходимости изучения и понимания процесса технологического прогресса. Авторы утверждают, что понимание эволюции технологий необходимо для полного использования их потенциала.
s modèles grand langage (LLM) et les modèles de diffusion tels que ChatGPT et Stable Diffusion ont montré un grand potentiel parce qu'ils ont été formés à tout le texte public et les images sur Internet, les rendant utiles pour un large éventail de tâches. Ces modèles peuvent révolutionner différents secteurs, de la santé à la finance et à l'éducation. Cependant, l'intégration de ces modèles dans des systèmes automatisés pose des problèmes considérables, comme l'obtention de résultats fiables.
Dans leur livre « Prompt Engineering for Generative AI FutureProof Inputs for Reliable AI Outputs », James Phoenix et Mike Taylor démontrent comment utiliser un ensemble de principes appelés James Phoenix prompt engineering, pour travailler efficacement avec l'IA. s auteurs montrent qu'en comprenant le processus d'évolution de la technologie, en développant un paradigme personnel de perception du processus technologique de développement des connaissances modernes et en étudiant la survie de l'humanité et l'unité dans un État en guerre, nous pouvons utiliser la puissance de la LLM et des modèles de diffusion pour résoudre des problèmes auparavant inadaptés à l'automatisation.
livre commence par étudier la nécessité d'étudier et de comprendre le processus de progrès technologique. s auteurs affirment que la compréhension de l'évolution des technologies est nécessaire pour exploiter pleinement leur potentiel.
Dans leur livre « Prompt Engineering for Generative AI FutureProof Inputs for Reliable AI Outputs », James Phoenix et Mike Taylor démontrent comment utiliser un ensemble de principes appelés James Phoenix prompt engineering, pour travailler efficacement avec l'IA. s auteurs montrent qu'en comprenant le processus d'évolution de la technologie, en développant un paradigme personnel de perception du processus technologique de développement des connaissances modernes et en étudiant la survie de l'humanité et l'unité dans un État en guerre, nous pouvons utiliser la puissance de la LLM et des modèles de diffusion pour résoudre des problèmes auparavant inadaptés à l'automatisation.
livre commence par étudier la nécessité d'étudier et de comprendre le processus de progrès technologique. s auteurs affirment que la compréhension de l'évolution des technologies est nécessaire pour exploiter pleinement leur potentiel.
modelos de lenguaje grande (LLM) y los modelos de difusión como ChatGPT y Stable Diffusion han mostrado un gran potencial porque han sido entrenados en todo el texto público e imágenes en línea, lo que los hace útiles para una amplia gama de tareas. Estos modelos pueden revolucionar diversas industrias, desde la salud hasta las finanzas y la educación. n embargo, la integración de estos modelos en sistemas automatizados plantea retos significativos, como la obtención de resultados fiables.
En su libro «Prompt Engineering for Generative AI FutureProof Inputs for Reliable AI Outputs», James Phoenix y Mike Taylor (Mike Taylor) demuestran cómo utilizar un conjunto de principios llamados ingeniería prompt para trabajar eficazmente con IA. autores demuestran que al comprender el proceso de evolución de la tecnología, desarrollar un paradigma personal de percepción del proceso tecnológico del desarrollo del conocimiento moderno y estudiar la supervivencia de la humanidad y la unidad en un Estado en guerra, podemos utilizar el poder de la LLM y los modelos de difusión para resolver problemas que antes no eran aptos para la automatización.
libro comienza explorando la necesidad de aprender y entender el proceso de progreso tecnológico. autores sostienen que la comprensión de la evolución de las tecnologías es necesaria para aprovechar plenamente su potencial.
En su libro «Prompt Engineering for Generative AI FutureProof Inputs for Reliable AI Outputs», James Phoenix y Mike Taylor (Mike Taylor) demuestran cómo utilizar un conjunto de principios llamados ingeniería prompt para trabajar eficazmente con IA. autores demuestran que al comprender el proceso de evolución de la tecnología, desarrollar un paradigma personal de percepción del proceso tecnológico del desarrollo del conocimiento moderno y estudiar la supervivencia de la humanidad y la unidad en un Estado en guerra, podemos utilizar el poder de la LLM y los modelos de difusión para resolver problemas que antes no eran aptos para la automatización.
libro comienza explorando la necesidad de aprender y entender el proceso de progreso tecnológico. autores sostienen que la comprensión de la evolución de las tecnologías es necesaria para aprovechar plenamente su potencial.
I modelli di grandi lingue (LLM) e i modelli di diffusione come e Difference hanno mostrato un grande potenziale perché sono stati addestrati a tutto il testo pubblico e le immagini online, rendendoli utili per una vasta gamma di attività. Questi modelli possono rivoluzionare diversi settori, dalla sanità alla finanza e all'istruzione. Tuttavia, l'integrazione di questi modelli in sistemi automatizzati pone problemi significativi, quali risultati affidabili.
Nel suo libro «Prompt Engineering for Generative AI», James Phoenix e Mike Taylor dimostrano come utilizzare un insieme di principi chiamati prompt engineering, per gestire l'IA in modo efficiente. Gli autori dimostrano che, capendo l'evoluzione della tecnologia, sviluppando un paradigma personale della percezione del processo tecnologico per lo sviluppo delle conoscenze moderne e studiando la sopravvivenza dell'umanità e l'unità in uno Stato in guerra, possiamo utilizzare il potere della LLM e dei modelli di diffusione per risolvere problemi precedentemente inutilizzabili.
Il libro inizia studiando la necessità di studiare e comprendere il processo di progresso tecnologico. Gli autori sostengono che comprendere l'evoluzione della tecnologia sia essenziale per sfruttare pienamente il loro potenziale.
Nel suo libro «Prompt Engineering for Generative AI», James Phoenix e Mike Taylor dimostrano come utilizzare un insieme di principi chiamati prompt engineering, per gestire l'IA in modo efficiente. Gli autori dimostrano che, capendo l'evoluzione della tecnologia, sviluppando un paradigma personale della percezione del processo tecnologico per lo sviluppo delle conoscenze moderne e studiando la sopravvivenza dell'umanità e l'unità in uno Stato in guerra, possiamo utilizzare il potere della LLM e dei modelli di diffusione per risolvere problemi precedentemente inutilizzabili.
Il libro inizia studiando la necessità di studiare e comprendere il processo di progresso tecnologico. Gli autori sostengono che comprendere l'evoluzione della tecnologia sia essenziale per sfruttare pienamente il loro potenziale.
Big Language Models (LLMs) und Diffusionsmodelle wie ChatGPT und Stable Diffusion haben großes Potenzial gezeigt, weil sie in allen öffentlichen Texten und Bildern im Internet geschult wurden, was sie für eine Vielzahl von Aufgaben nützlich macht. Diese Modelle haben das Potenzial, eine Vielzahl von Branchen zu revolutionieren, von Gesundheitswesen über Finanzen bis hin zu Bildung. Die Integration dieser Modelle in automatisierte Systeme stellt jedoch erhebliche Herausforderungen dar, wie z.B. die Erzielung zuverlässiger Ergebnisse.
In ihrem Buch „Prompt Engineering for Generative AI FutureProof Inputs for Reliable AI Outputs“ zeigen James Phoenix und Mike Taylor, wie man eine Reihe von Prinzipien namens Prompt Engineering nutzt, um effektiv mit KI zu arbeiten Die Autoren zeigen, dass wir durch das Verständnis des technologischen Evolutionsprozesses, die Entwicklung eines persönlichen Paradigmas für die Wahrnehmung des technologischen Prozesses der Entwicklung des modernen Wissens und das Studium des Überlebens der Menschheit und der Einheit in einem kriegsführenden Staat die Macht von LLM und Diffusionsmodellen nutzen können, um Probleme zu lösen, die zuvor für die Automatisierung ungeeignet waren.
Das Buch beginnt mit der Untersuchung der Notwendigkeit, den Prozess des technologischen Fortschritts zu studieren und zu verstehen. Die Autoren argumentieren, dass das Verständnis der Technologieentwicklung notwendig ist, um ihr Potenzial voll auszuschöpfen.
In ihrem Buch „Prompt Engineering for Generative AI FutureProof Inputs for Reliable AI Outputs“ zeigen James Phoenix und Mike Taylor, wie man eine Reihe von Prinzipien namens Prompt Engineering nutzt, um effektiv mit KI zu arbeiten Die Autoren zeigen, dass wir durch das Verständnis des technologischen Evolutionsprozesses, die Entwicklung eines persönlichen Paradigmas für die Wahrnehmung des technologischen Prozesses der Entwicklung des modernen Wissens und das Studium des Überlebens der Menschheit und der Einheit in einem kriegsführenden Staat die Macht von LLM und Diffusionsmodellen nutzen können, um Probleme zu lösen, die zuvor für die Automatisierung ungeeignet waren.
Das Buch beginnt mit der Untersuchung der Notwendigkeit, den Prozess des technologischen Fortschritts zu studieren und zu verstehen. Die Autoren argumentieren, dass das Verständnis der Technologieentwicklung notwendig ist, um ihr Potenzial voll auszuschöpfen.
''
Büyük Dil Modelleri (LLM'ler) ve ChatGPT ve Stable Diffusion gibi difüzyon modelleri, tüm genel metin ve görüntüler üzerinde çevrimiçi olarak eğitildikleri için çok çeşitli görevler için yararlı olduklarından büyük bir potansiyel göstermiştir. Bu modeller, sağlıktan finansa ve eğitime kadar değişen sektörlerde devrim yapma potansiyeline sahiptir. Bununla birlikte, bu modelleri otomatik sistemlere entegre etmek, güvenilir sonuçlar elde etmek gibi önemli zorluklar doğurmaktadır.
Prompt Engineering for Generative AI FutureProof Input for Reliable AI Outputs adlı kitaplarında, James Phoenix ve Mike Taylor, yapay zeka ile etkili bir şekilde çalışmak için hızlı mühendislik adı verilen bir dizi ilkenin nasıl kullanılacağını gösteriyor Yazarlar, teknolojinin evrimini anlayarak, Modern bilginin gelişiminin teknolojik sürecinin algılanması için kişisel bir paradigma geliştirmek ve savaşan bir devlette insanlığın ve birliğin hayatta kalmasını incelemek, Daha önce otomasyon için uygun olmayan sorunları çözmek için LLM ve difüzyon modellerinin gücünü kullanabiliriz. Kitap, teknolojik ilerleme sürecini öğrenme ve anlama ihtiyacını inceleyerek başlar. Teknolojinin evrimini anlamak, potansiyelini tam olarak kullanmak için gereklidir, yazarlar savunuyorlar.
Prompt Engineering for Generative AI FutureProof Input for Reliable AI Outputs adlı kitaplarında, James Phoenix ve Mike Taylor, yapay zeka ile etkili bir şekilde çalışmak için hızlı mühendislik adı verilen bir dizi ilkenin nasıl kullanılacağını gösteriyor Yazarlar, teknolojinin evrimini anlayarak, Modern bilginin gelişiminin teknolojik sürecinin algılanması için kişisel bir paradigma geliştirmek ve savaşan bir devlette insanlığın ve birliğin hayatta kalmasını incelemek, Daha önce otomasyon için uygun olmayan sorunları çözmek için LLM ve difüzyon modellerinin gücünü kullanabiliriz. Kitap, teknolojik ilerleme sürecini öğrenme ve anlama ihtiyacını inceleyerek başlar. Teknolojinin evrimini anlamak, potansiyelini tam olarak kullanmak için gereklidir, yazarlar savunuyorlar.
أظهرت نماذج اللغات الكبيرة (LLMs) ونماذج الانتشار مثل ChatGPT و Stable Diffusion إمكانات كبيرة لأنها تم تدريبها على جميع النصوص والصور العامة عبر الإنترنت، مما يجعلها مفيدة لمجموعة واسعة من المهام. هذه النماذج لديها القدرة على إحداث ثورة في الصناعات التي تتراوح من الرعاية الصحية إلى التمويل إلى التعليم. ومع ذلك، فإن دمج هذه النماذج في الأنظمة الآلية يطرح تحديات كبيرة، مثل الحصول على نتائج موثوقة.
في كتابهم الهندسة الفورية لمدخلات مستقبل الذكاء الاصطناعي المولدة لمخرجات الذكاء الاصطناعي الموثوقة، يوضح جيمس فينيكس ومايك تايلور كيفية استخدام مجموعة من المبادئ تسمى الهندسة السريعة للعمل بفعالية مع الذكاء الاصطناعي. يُظهر المؤلفون أنه من خلال فهم تطور التكنولوجيا، وضع نموذج شخصي لتصور العملية التكنولوجية لتطور المعرفة الحديثة ودراسة بقاء البشرية والوحدة في حالة حرب، يمكننا استخدام قوة LLM ونماذج الانتشار لحل المشكلات التي لم تكن مناسبة في السابق للأتمتة. يبدأ الكتاب بدراسة الحاجة إلى تعلم وفهم عملية التقدم التكنولوجي. يجادل المؤلفون بأن فهم تطور التكنولوجيا ضروري للاستفادة الكاملة من إمكاناتها.
في كتابهم الهندسة الفورية لمدخلات مستقبل الذكاء الاصطناعي المولدة لمخرجات الذكاء الاصطناعي الموثوقة، يوضح جيمس فينيكس ومايك تايلور كيفية استخدام مجموعة من المبادئ تسمى الهندسة السريعة للعمل بفعالية مع الذكاء الاصطناعي. يُظهر المؤلفون أنه من خلال فهم تطور التكنولوجيا، وضع نموذج شخصي لتصور العملية التكنولوجية لتطور المعرفة الحديثة ودراسة بقاء البشرية والوحدة في حالة حرب، يمكننا استخدام قوة LLM ونماذج الانتشار لحل المشكلات التي لم تكن مناسبة في السابق للأتمتة. يبدأ الكتاب بدراسة الحاجة إلى تعلم وفهم عملية التقدم التكنولوجي. يجادل المؤلفون بأن فهم تطور التكنولوجيا ضروري للاستفادة الكاملة من إمكاناتها.
