
BOOKS - Effective XGBoost Optimizing, Tuning, Understanding, and Deploying Classifica...

Effective XGBoost Optimizing, Tuning, Understanding, and Deploying Classification Models
Author: Matt Harrison
Year: 2023
Format: PDF
File size: 28.9 MB
Language: ENG
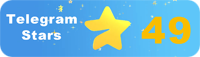
Year: 2023
Format: PDF
File size: 28.9 MB
Language: ENG
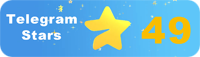
Book Description: Effective XGBoost: Optimizing Tuning, Understanding, and Deploying Classification Models In today's fast-paced world, technology is constantly evolving, and it's essential to stay ahead of the curve to remain relevant. One of the most popular machine learning algorithms used in data science today is XGBoost, a powerful tool for handling large datasets, missing values, and nonlinear relationships. In this comprehensive guide, you'll learn everything you need to know to become an expert in XGBoost, from the basics of classification tasks to advanced techniques for optimizing and deploying your models. Understanding XGBoost XGBoost is both a library and a particular gradient boosted trees (GBT) algorithm that utilizes ensembling to build a very strong machine learning algorithm by combining many weaker algorithms. You'll start with the basics, learning how to prepare your data, select the right features, and train your model. As you progress, you'll explore advanced techniques for optimizing your models, including hyperparameter tuning, early stopping, and ensemble methods. Preparing Your Data - Machine learning for tabular data is still a very hands-on artisanal process, and a big part of what makes a great tabular data ML model has to do with proper data preparation and feature engineering.
Эффективный XGBoost: оптимизация настройки, понимания и развертывания классификационных моделей В современном быстро развивающемся мире технологии постоянно развиваются, и важно быть на опережение, чтобы оставаться актуальным. Одним из самых популярных алгоритмов машинного обучения, используемых в науке о данных сегодня, является XGBoost, мощный инструмент для обработки больших наборов данных, отсутствующих значений и нелинейных отношений. В этом полном руководстве вы узнаете все, что вам нужно знать, чтобы стать экспертом в XGBoost, от основ задач классификации до передовых методов оптимизации и развертывания ваших моделей. Понимание XGBoost XGBoost - это как библиотека, так и конкретный алгоритм, усиленный градиентом деревьев (GBT), который использует ассемблирование для построения очень сильного алгоритма машинного обучения, комбинируя много более слабых алгоритмов. Вы начнете с основ, научитесь готовить данные, выберете нужные функции и обучите свою модель. По мере развития вы будете изучать передовые методы оптимизации моделей, включая настройку гиперпараметров, раннюю остановку и методы ансамбля. Подготовка данных - машинное обучение для табличных данных по-прежнему является очень практичным кустарным процессом, и большая часть того, что делает отличную модель ML табличных данных, связана с правильной подготовкой данных и разработкой функций.
Efficace XGBoost : optimiser la personnalisation, la compréhension et le déploiement des modèles de classification Dans le monde en évolution rapide d'aujourd'hui, les technologies évoluent constamment et il est important d'être en avance pour rester à jour. L'un des algorithmes d'apprentissage automatique les plus populaires utilisés dans la science des données aujourd'hui est XGBoost, un outil puissant pour traiter de grands ensembles de données, des valeurs manquantes et des relations non linéaires. Dans ce guide complet, vous apprendrez tout ce que vous devez savoir pour devenir un expert en XGBoost, des bases des tâches de classification aux meilleures pratiques pour optimiser et déployer vos modèles. Comprendre XGBoost XGBoost est à la fois une bibliothèque et un algorithme spécifique renforcé par un gradient d'arbres (GBT) qui utilise l'assemblage pour construire un algorithme d'apprentissage automatique très fort, combinant de nombreux algorithmes plus faibles. Vous commencerez par les bases, apprendrez à préparer les données, choisirez les bonnes fonctions et formerez votre modèle. Au fur et à mesure que vous progresserez, vous apprendrez les meilleures techniques d'optimisation des modèles, y compris l'ajustement des hyperparamètres, l'arrêt précoce et les méthodes d'ensemble. Préparation des données - l'apprentissage automatique pour les données tabulaires est toujours un processus artisanal très pratique, et une grande partie de ce qui fait un excellent modèle ML de données tabulaires est liée à la préparation correcte des données et au développement des fonctions.
XGBoost eficiente: optimizar la personalización, la comprensión y el despliegue de los modelos de clasificación En el mundo de hoy en desarrollo, la tecnología evoluciona constantemente y es importante estar a la vanguardia para mantenerse al día. Uno de los algoritmos de aprendizaje automático más populares utilizados en la ciencia de datos en la actualidad es XGBoost, una poderosa herramienta para procesar grandes conjuntos de datos, valores ausentes y relaciones no lineales. En esta guía completa aprenderás todo lo que necesitas saber para convertirte en un experto en XGBoost, desde los fundamentos de las tareas de clasificación hasta las técnicas avanzadas de optimización e implementación de tus modelos. La comprensión de XGBoost XGBoost es tanto una biblioteca como un algoritmo específico reforzado por un gradiente de árbol (GBT) que utiliza ensamblaje para construir un algoritmo de aprendizaje automático muy fuerte, combinando muchos algoritmos más débiles. Empezarás con lo básico, aprenderás a preparar los datos, elegirás las funciones que quieras y enseñarás tu modelo. A medida que avanza, aprenderá técnicas avanzadas de optimización de modelos, incluyendo ajuste de hiperparámetros, parada temprana y técnicas de ensamble. Preparación de datos: el aprendizaje automático para datos tabulares sigue siendo un proceso artesanal muy práctico, y gran parte de lo que hace un excelente modelo ML de datos tabulares tiene que ver con la correcta preparación de datos y el desarrollo de funciones.
Efficient XGBoost: Optimierung der Konfiguration, des Verständnisses und des Einsatzes von Klassifikationsmodellen In der heutigen schnelllebigen Welt entwickelt sich die Technologie ständig weiter und es ist wichtig, der Zeit voraus zu sein, um relevant zu bleiben. Einer der beliebtesten Algorithmen für maschinelles rnen, die heute in der Datenwissenschaft verwendet werden, ist XGBoost, ein leistungsfähiges Werkzeug zur Verarbeitung großer Datensätze, fehlender Werte und nichtlinearer Beziehungen. In diesem vollständigen itfaden erfahren e alles, was e wissen müssen, um ein Experte für XGBoost zu werden, von den Grundlagen der Klassifizierungsprobleme bis hin zu Best Practices für die Optimierung und Bereitstellung Ihrer Modelle. XGBoost verstehen XGBoost ist sowohl eine Bibliothek als auch ein spezifischer Algorithmus, der durch den Baumgradienten (GBT) verstärkt wird und Assembling verwendet, um einen sehr starken Algorithmus für maschinelles rnen aufzubauen, indem viele schwächere Algorithmen kombiniert werden. e beginnen mit den Grundlagen, lernen, wie e Daten vorbereiten, die gewünschten Funktionen auswählen und Ihr Modell trainieren. Während e sich weiterentwickeln, lernen e fortgeschrittene Methoden zur Optimierung von Modellen, einschließlich der Einstellung von Hyperparametern, frühem Stillstand und Ensembletechniken. Datenaufbereitung - Maschinelles rnen für Tabellendaten ist immer noch ein sehr praktischer handwerklicher Prozess, und ein Großteil dessen, was ein ausgezeichnetes ML-Modell von Tabellendaten ausmacht, hängt mit der richtigen Datenaufbereitung und Funktionsentwicklung zusammen.
''
Verimli XGBoost: Sınıflandırma Modellerinin Kurulumu, Anlaşılması ve Dağıtımının Optimize Edilmesi Günümüzün hızlı dünyasında, teknoloji sürekli gelişmektedir ve alakalı kalmak için eğrinin önünde kalmak önemlidir. Günümüzde veri biliminde kullanılan en popüler makine öğrenme algoritmalarından biri, büyük veri kümelerini, eksik değerleri ve doğrusal olmayan ilişkileri işlemek için güçlü bir araç olan XGBoost'tur. Bu eksiksiz kılavuzda, sınıflandırma görevlerinin temellerinden modellerinizi optimize etmek ve dağıtmak için en iyi uygulamalara kadar bir XGBoost uzmanı olmak için bilmeniz gereken her şeyi öğreneceksiniz. XGBoost'u anlamak XGBoost, hem bir kütüphane hem de birçok zayıf algoritmayı birleştirerek çok güçlü bir makine öğrenme algoritması oluşturmak için montajı kullanan somut bir ağaç gradyanı geliştirilmiş algoritmadır (GBT). Temel bilgilerle başlayacak, verilerin nasıl hazırlanacağını öğrenecek, ihtiyacınız olan işlevleri seçecek ve modelinizi eğiteceksiniz. İlerledikçe, hiper parametre ayarı, erken durdurma ve topluluk teknikleri de dahil olmak üzere gelişmiş model optimizasyon tekniklerini öğreneceksiniz. Veri hazırlama - Tablo verileri için makine öğrenimi hala çok pratik bir zanaatkar süreçtir ve tablo verilerinin mükemmel bir ML modelini oluşturan şeylerin çoğu, uygun veri hazırlama ve özellik geliştirme ile ilgilidir.
كفاءة XGBoost: تحسين إعداد وفهم ونشر نماذج التصنيف في عالم اليوم سريع الخطى، تتطور التكنولوجيا باستمرار، ومن المهم البقاء في طليعة المنحنى للبقاء على صلة. واحدة من أشهر خوارزميات التعلم الآلي المستخدمة في علوم البيانات اليوم هي XGBoost، وهي أداة قوية للتعامل مع مجموعات البيانات الكبيرة والقيم المفقودة والعلاقات غير الخطية. في هذا الدليل الكامل، ستتعلم كل ما تحتاج إلى معرفته لتصبح خبيرًا في XGBost، من أساسيات مهام التصنيف إلى أفضل الممارسات لتحسين نماذجك ونشرها. إن فهم XGBoost XGBoost هو مكتبة وخوارزمية خرسانية معززة بالتدرج (GBT) تستخدم التجميع لبناء خوارزمية تعلم آلي قوية جدًا من خلال الجمع بين العديد من الخوارزميات الأضعف. ستبدأ بالأساسيات وتتعلم كيفية إعداد البيانات واختيار الوظائف التي تحتاجها وتدريب نموذجك. مع تقدمك، ستتعلم تقنيات تحسين النماذج المتقدمة، بما في ذلك ضبط هايبرباراميتر، والتوقف المبكر، وتقنيات المجموعة. إعداد البيانات - لا يزال التعلم الآلي للبيانات المجدولة عملية حرفية عملية للغاية والكثير مما يجعل نموذج ML ممتازًا للبيانات المجدولة مرتبط بإعداد البيانات المناسبة وتطوير الميزات.
