
BOOKS - PROGRAMMING - Effective Machine Learning Teams Best Practices for ML Practiti...

Effective Machine Learning Teams Best Practices for ML Practitioners (Fifth Early Release)
Author: David Tan, Ada Leung
Year: 2023-07-18
Format: PDF | EPUB | MOBI
File size: 10.1 MB
Language: ENG
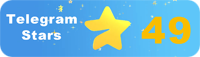
Year: 2023-07-18
Format: PDF | EPUB | MOBI
File size: 10.1 MB
Language: ENG
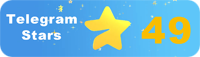
Effective Machine Learning Teams Best Practices for ML Practitioners Fifth Early Release In today's fast-paced technological landscape, it is essential to understand the process of technology evolution and its impact on humanity's survival. The book "Effective Machine Learning Teams" by David Tan and Ada Leung provides valuable insights into the best practices for machine learning (ML) practitioners to accelerate the delivery of ML solutions. As technology continues to advance at an unprecedented rate, it is crucial to develop a personal paradigm for perceiving the technological process of developing modern knowledge. This paradigm will serve as the basis for the survival of humanity and the unification of people in a warring state. The book focuses on bridging the gap between data science and Lean software delivery, offering practical and simple techniques that data scientists and ML engineers can apply to improve their effectiveness in ML projects. The authors draw on their extensive experience across multiple real-world data and ML projects to help teams avoid common traps in the ML world, enabling them to iterate more quickly and reliably. By mastering these techniques, data scientists and ML engineers can overcome friction and experience flow when delivering machine learning solutions. The book is divided into four parts, each addressing a critical aspect of ML project delivery: Part 1: Understanding the Fundamentals of ML Project Delivery In this part, the authors provide a comprehensive overview of the ML development process, highlighting the importance of understanding the technology evolution and its impact on humanity's survival.
Эффективные команды машинного обучения Лучшие практики для практиков ML Пятый ранний выпуск В современном быстро развивающемся технологическом ландшафте важно понимать процесс эволюции технологий и его влияние на выживание человечества. Книга «Эффективные команды машинного обучения» Дэвида Тана и Ады Люн дает ценную информацию о лучших практикующих практикующих практикующих специалистов по машинному обучению (ML) для ускорения предоставления ML-решений. Поскольку технологии продолжают развиваться беспрецедентными темпами, крайне важно разработать личную парадигму восприятия технологического процесса развития современных знаний. Эта парадигма послужит основой для выживания человечества и объединения людей в воюющем государстве. Книга посвящена преодолению разрыва между наукой о данных и предоставлением программного обеспечения an, предлагая практические и простые методы, которые специалисты по данным и инженеры ML могут применять для повышения их эффективности в проектах ML. Авторы опираются на свой обширный опыт работы с несколькими реальными данными и проектами ML, чтобы помочь командам избежать распространенных ловушек в мире ML, что позволяет им быстрее и надежнее выполнять итерации. Осваивая эти методы, специалисты по данным и инженеры ML могут преодолеть трение и ощутить поток при поставке решений для машинного обучения. Книга разделена на четыре части, каждая из которых посвящена критическому аспекту реализации проекта ML: Часть 1: Понимание основ реализации проекта ML В этой части авторы дают всесторонний обзор процесса разработки ML, подчеркивая важность понимания эволюция технологии и ее влияние на выживание человечества.
Des équipes d'apprentissage automatique efficaces Meilleures pratiques pour les praticiens ML Cinquième numéro Dans le paysage technologique en évolution rapide d'aujourd'hui, il est important de comprendre le processus d'évolution de la technologie et son impact sur la survie de l'humanité. livre « Des équipes d'apprentissage automatique efficaces » de David Tan et Ada ung fournit des informations précieuses sur les meilleurs praticiens praticiens de l'apprentissage automatique (ML) pour accélérer la fourniture de solutions ML. Alors que la technologie continue d'évoluer à un rythme sans précédent, il est essentiel d'élaborer un paradigme personnel pour la perception du processus technologique du développement des connaissances modernes. Ce paradigme servira de base à la survie de l'humanité et à l'unification des hommes dans un État en guerre. livre vise à combler le fossé entre la science des données et la fourniture de logiciels an, en proposant des méthodes pratiques et simples que les spécialistes des données et les ingénieurs ML peuvent appliquer pour améliorer leur efficacité dans les projets ML. s auteurs s'appuient sur leur vaste expérience avec plusieurs données réelles et projets ML pour aider les équipes à éviter les pièges courants dans le monde ML, ce qui leur permet d'effectuer des itérations plus rapidement et plus efficacement. En maîtrisant ces méthodes, les experts en données et les ingénieurs ML peuvent surmonter les frottements et ressentir le flux lors de la fourniture de solutions d'apprentissage automatique. livre est divisé en quatre parties, chacune consacrée à l'aspect critique de la mise en œuvre du projet ML : Partie 1 : Comprendre les bases de la mise en œuvre du projet ML Dans cette partie, les auteurs donnent un aperçu complet du processus de développement du ML, soulignant l'importance de comprendre l'évolution de la technologie et son impact sur la survie de l'humanité.
Equipos eficientes de aprendizaje automático Mejores prácticas para profesionales ML Quinto lanzamiento temprano En el panorama tecnológico en rápida evolución actual, es importante comprender el proceso de evolución de la tecnología y su impacto en la supervivencia de la humanidad. libro «Eficient Machine arning Team» de David Tan y Ada ung proporciona información valiosa sobre los mejores practicantes practicantes de machine learning (ML) para acelerar la entrega de soluciones de ML. A medida que la tecnología continúa evolucionando a un ritmo sin precedentes, es esencial desarrollar un paradigma personal para percibir el proceso tecnológico del desarrollo del conocimiento moderno. Este paradigma servirá de base para la supervivencia de la humanidad y la unificación de los seres humanos en un Estado en guerra. libro trata de cerrar la brecha entre la ciencia de datos y la provisión de software, ofreciendo métodos prácticos y sencillos que los expertos en datos e ingenieros de ML pueden aplicar para mejorar su eficiencia en los proyectos de ML. autores confían en su amplia experiencia con varios datos reales y proyectos de ML para ayudar a los equipos a evitar trampas comunes en el mundo de ML, lo que les permite realizar iteraciones de forma más rápida y fiable. Al dominar estas técnicas, los expertos en datos e ingenieros de ML pueden superar la fricción y sentir el flujo al suministrar soluciones de aprendizaje automático. libro se divide en cuatro partes, cada una dedicada al aspecto crítico de la implementación del proyecto ML: Parte 1: Comprender los fundamentos de la implementación del proyecto ML En esta parte, los autores ofrecen una visión completa del proceso de desarrollo del ML, destacando la importancia de comprender la evolución de la tecnología y su impacto en la supervivencia de la humanidad.
Equipes eficientes de aprendizagem de máquinas As melhores práticas para os praticantes de ML Quinta Edição Antecipada No atual panorama tecnológico em rápida evolução é importante compreender o processo de evolução da tecnologia e seus efeitos na sobrevivência humana. O livro «Equipes de Aprendizagem de Máquinas Eficientes», de David Tang e Ada ung, fornece informações valiosas sobre os melhores praticantes de aprendizagem de máquina (ML) para acelerar o fornecimento de soluções ML. Como a tecnologia continua a evoluir a um ritmo sem precedentes, é crucial desenvolver um paradigma pessoal para a percepção do processo tecnológico de desenvolvimento do conhecimento moderno. Este paradigma servirá de base para a sobrevivência da humanidade e para a união das pessoas num estado em guerra. O livro é dedicado a superar o fosso entre a ciência dos dados e o fornecimento de software an, oferecendo técnicas práticas e simples que os especialistas em dados e engenheiros da ML podem aplicar para melhorar sua eficiência em projetos ML. Os autores se baseiam em sua vasta experiência com vários dados reais e projetos ML para ajudar as equipes a evitar armadilhas comuns no mundo ML, permitindo-lhes realizar iterações mais rápidas e confiáveis. Ao aprender estes métodos, os especialistas em dados e os engenheiros da ML podem superar o atrito e sentir o fluxo ao fornecer soluções de aprendizado de máquina. O livro é dividido em quatro partes, cada uma sobre o aspecto crítico do projeto ML: Parte 1: Compreensão dos fundamentos do projeto ML Nesta parte, os autores dão uma visão completa do processo de desenvolvimento do ML, destacando a importância de compreender a evolução da tecnologia e seus efeitos na sobrevivência humana.
Efficaci team di apprendimento automatico migliori pratiche per i praticanti di ML Quinta edizione precoce In un panorama tecnologico in continua evoluzione, è importante comprendere l'evoluzione della tecnologia e il suo impatto sulla sopravvivenza dell'umanità. I team di apprendimento automatico di David Tang e Ada ung forniscono preziose informazioni sui migliori praticanti di apprendimento automatico (ML) per accelerare la fornitura di soluzioni ML. Poiché la tecnologia continua a crescere a un ritmo senza precedenti, è fondamentale sviluppare un paradigma personale per la percezione del processo tecnologico di sviluppo della conoscenza moderna. Questo paradigma sarà la base per la sopravvivenza dell'umanità e per l'unione delle persone in uno stato in guerra. Il libro è dedicato a colmare il divario tra la scienza dei dati e la fornitura di software an, offrendo metodi pratici e semplici che gli esperti di dati e gli ingegneri di ML possono applicare per migliorarne l'efficacia nei progetti ML. Gli autori si basano sulla loro vasta esperienza con più dati reali e progetti ML per aiutare i team a evitare trappole comuni nel mondo ML, consentendo loro di eseguire iterazioni più rapidamente e affidabilmente. Imparando queste tecniche, gli esperti di dati e gli ingegneri ML possono superare l'attrito e percepire il flusso quando forniscono soluzioni di apprendimento automatico. Il libro è suddiviso in quattro parti, ognuna dedicata all'aspetto critico del progetto ML: Parte 1: Comprendere le basi del progetto ML In questa parte, gli autori forniscono una panoramica completa del processo di sviluppo di ML, sottolineando l'importanza di comprendere l'evoluzione della tecnologia e il suo impatto sulla sopravvivenza dell'umanità.
Effektive Teams für maschinelles rnen Best Practices für Praktiker ML Fünftes Early Release In der heutigen schnelllebigen Technologielandschaft ist es wichtig, den technologischen Evolutionsprozess und seine Auswirkungen auf das Überleben der Menschheit zu verstehen. Das Buch „Effective Machine arning Teams“ von David Tan und Ada ung liefert wertvolle Einblicke in Best Practice Practice Practitioner von Machine arning (ML) -Spezialisten, um die Bereitstellung von ML-Lösungen zu beschleunigen. Da sich die Technologie in einem beispiellosen Tempo weiterentwickelt, ist es von entscheidender Bedeutung, ein persönliches Paradigma für die Wahrnehmung des technologischen Prozesses der Entwicklung des modernen Wissens zu entwickeln. Dieses Paradigma wird als Grundlage für das Überleben der Menschheit und die Vereinigung der Menschen in einem kriegführenden Staat dienen. Das Buch konzentriert sich auf die Überbrückung der Lücke zwischen Data Science und der Bereitstellung von an-Software und bietet praktische und einfache Methoden, die Datenwissenschaftler und ML-Ingenieure anwenden können, um ihre Effizienz in ML-Projekten zu verbessern. Die Autoren greifen auf ihre umfangreiche Erfahrung mit mehreren realen ML-Daten und -Projekten zurück, um Teams dabei zu helfen, häufige Fallen in der ML-Welt zu vermeiden, sodass sie schneller und zuverlässiger iterieren können. Durch die Beherrschung dieser Methoden können Datenwissenschaftler und ML-Ingenieure die Reibung überwinden und den Fluss bei der Bereitstellung von Lösungen für maschinelles rnen spüren. Das Buch ist in vier Teile gegliedert, die sich jeweils mit einem kritischen Aspekt der ML-Projektumsetzung befassen: Teil 1: Verständnis der Grundlagen der ML-Projektumsetzung In diesem Teil geben die Autoren einen umfassenden Überblick über den ML-Entwicklungsprozess und betonen, wie wichtig es ist, die Evolution der Technologie und ihre Auswirkungen auf das Überleben der Menschheit zu verstehen.
''
Etkili Makine Öğrenimi Ekipleri ML Uygulayıcıları için En İyi Uygulamalar Beşinci Erken Baskı Günümüzün hızla gelişen teknolojik ortamında, teknolojinin evrimini ve insanın hayatta kalması üzerindeki etkisini anlamak önemlidir. David Tan ve Ada ung tarafından hazırlanan "Effective Machine arning Teams" kitabı, ML çözümlerinin sunulmasını hızlandırmak için en iyi makine öğrenimi (ML) uygulayıcılarına değerli bilgiler sunmaktadır. Teknoloji benzeri görülmemiş bir hızda gelişmeye devam ettikçe, modern bilginin geliştirilmesinin teknolojik sürecinin algılanması için kişisel bir paradigma geliştirmek zorunludur. Bu paradigma, insanlığın hayatta kalması ve insanların savaşan bir durumda birleşmesi için temel oluşturacaktır. Kitap, veri bilimi ile an yazılımının sağlanması arasındaki boşluğu kapatmaya odaklanarak, ML veri bilimcilerinin ve mühendislerinin ML projelerinde verimliliklerini artırmak için uygulayabilecekleri pratik ve basit yöntemler sunuyor. Yazarlar, ekiplerin ML dünyasındaki yaygın tuzaklardan kaçınmasına yardımcı olmak için çeşitli gerçek dünya ML verileri ve projeleriyle ilgili kapsamlı deneyimlerinden yararlanarak, daha hızlı ve daha güvenilir bir şekilde yinelemelerini sağlar. Bu yöntemlere hakim olan ML veri bilimcileri ve mühendisleri, makine öğrenimi çözümleri sunarken sürtünmenin üstesinden gelebilir ve akışı hissedebilir. Kitap, her biri ML projesinin uygulanmasının kritik bir yönüne ayrılmış dört bölüme ayrılmıştır: Bölüm 1: ML projesinin uygulanmasının temellerini anlama Bu bölümde, yazarlar teknolojinin evrimini ve insanlığın hayatta kalması üzerindeki etkisini anlamanın önemini vurgulayarak ML geliştirme sürecine kapsamlı bir genel bakış sunmaktadır.
فرق التعلم الآلي الفعالة أفضل الممارسات لممارسي ML الإصدار الخامس المبكر في المشهد التكنولوجي سريع التطور اليوم، من المهم فهم تطور التكنولوجيا وتأثيرها على بقاء الإنسان. يقدم كتاب «فرق التعلم الآلي الفعالة» من تأليف David Tan و Ada ung رؤى قيمة حول أفضل ممارسي التعلم الآلي (ML) لتسريع تقديم حلول ML. ومع استمرار تطور التكنولوجيا بوتيرة لم يسبق لها مثيل، لا بد من وضع نموذج شخصي لتصور العملية التكنولوجية لتطوير المعرفة الحديثة. وسيكون هذا النموذج أساسا لبقاء البشرية وتوحيد الشعوب في دولة متحاربة. يركز الكتاب على سد الفجوة بين علم البيانات وتوفير برامج an، وتقديم طرق عملية وبسيطة يمكن لعلماء بيانات ML والمهندسين تطبيقها لتحسين كفاءتهم في مشاريع ML. يعتمد المؤلفون على تجربتهم الواسعة مع العديد من بيانات ومشاريع ML في العالم الحقيقي لمساعدة الفرق على تجنب المزالق الشائعة في عالم ML، مما يسمح لهم بالتكرار بشكل أسرع وأكثر موثوقية. من خلال إتقان هذه الأساليب، يمكن لعلماء بيانات ML والمهندسين التغلب على الاحتكاك والشعور بالتدفق عند تقديم حلول التعلم الآلي. ينقسم الكتاب إلى أربعة أجزاء، كل منها مخصص لجانب حاسم في تنفيذ مشروع ML: في هذا الجزء، يقدم المؤلفون لمحة عامة شاملة عن عملية تطوير القانون النموذجي، مؤكدين على أهمية فهم تطور التكنولوجيا وأثرها على بقاء البشرية.
