
BOOKS - Multi-Agent Reinforcement Learning Foundations and Modern Approaches

Multi-Agent Reinforcement Learning Foundations and Modern Approaches
Author: Stefano V. Albrecht, Filippos Christianos, Lukas Sch?fer
Year: December 17, 2024
Format: EPUB
File size: 14.3 MB
Language: ENG
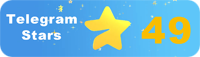
Year: December 17, 2024
Format: EPUB
File size: 14.3 MB
Language: ENG
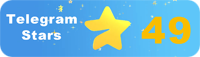
MultiAgent Reinforcement Learning Foundations and Modern Approaches The book "MultiAgent Reinforcement Learning Foundations and Modern Approaches" is a comprehensive guide to understanding the principles and applications of multi-agent reinforcement learning, a rapidly growing field that combines techniques from artificial intelligence, game theory, and control theory to solve complex problems in various domains. The book provides a thorough overview of the foundational concepts, algorithms, and applications of multi-agent reinforcement learning, making it an essential resource for researchers, students, and practitioners working in this area. The book begins by introducing the fundamental concepts of reinforcement learning and its extensions to multi-agent settings, where multiple agents interact with each other and learn to optimize their behaviors based on their observations and rewards. It covers the key challenges in multi-agent reinforcement learning, such as scalability, non-stationarity, and adversarial behavior, and discusses the different approaches to addressing these challenges. The book then delves into the foundational topics of multi-agent reinforcement learning, including the concept of a Nash equilibrium, the role of communication and cooperation, and the use of attention mechanisms to focus on relevant information. It also explores modern approaches to multi-agent reinforcement learning, such as deep reinforcement learning, off-policy learning, and transfer learning, and discusses their applications in areas like robotics, autonomous vehicles, and smart homes. One of the unique aspects of the book is its emphasis on the need to study and understand the process of technology evolution.
MultiAgent Reinforcement arning Foundations and Modern Approaches Книга «MultiAgent Reinforcement arning Foundations and Modern Approaches» - это всеобъемлющее руководство по пониманию принципов и приложений многоагентного обучения подкреплению, быстро развивающейся области, которая сочетает в себе методы искусственного интеллекта, теории игр и теории управления для решения сложных задач в различных областях. Книга содержит подробный обзор основополагающих концепций, алгоритмов и приложений многоагентного обучения с подкреплением, что делает его важным ресурсом для исследователей, студентов и практиков, работающих в этой области. Книга начинается с введения фундаментальных концепций обучения с подкреплением и его расширения до многоагентных настроек, где несколько агентов взаимодействуют друг с другом и учатся оптимизировать свое поведение на основе своих наблюдений и вознаграждений. Он охватывает ключевые проблемы многоагентного обучения с подкреплением, такие как масштабируемость, нестационарность и состязательное поведение, и обсуждает различные подходы к решению этих проблем. Затем книга углубляется в основополагающие темы многоагентного обучения с подкреплением, включая концепцию равновесия Нэша, роль общения и сотрудничества, а также использование механизмов внимания, чтобы сосредоточиться на соответствующей информации. В нем также рассматриваются современные подходы к многоагентному обучению с подкреплением, такие как глубокое обучение с подкреплением, обучение вне политики и обучение с передачей, а также обсуждаются их приложения в таких областях, как робототехника, автономные транспортные средства и умные дома. Один из уникальных аспектов книги - акцент на необходимости изучения и понимания процесса эволюции технологий.
''
