
BOOKS - Shallow Learning vs. Deep Learning A Practical Guide for Machine Learning Sol...

Shallow Learning vs. Deep Learning A Practical Guide for Machine Learning Solutions
Author: Omer Faruk Ertugrul, Josep M Guerrero, Musa Yilmaz
Year: 2024
Format: PDF | EPUB
File size: 45.5 MB
Language: ENG
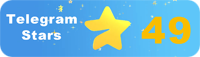
Year: 2024
Format: PDF | EPUB
File size: 45.5 MB
Language: ENG
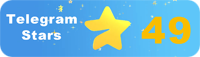
The book "Shallow Learning vs Deep Learning A Practical Guide for Machine Learning Solutions" explores the differences between shallow learning and deep learning, two fundamental approaches to machine learning that have revolutionized the field of artificial intelligence. The author, a renowned expert in the field, provides a comprehensive overview of these techniques, explaining their strengths and weaknesses, and offering practical guidance on how to choose the right approach for your specific needs. The book begins by defining the key concepts of shallow and deep learning, and discussing the historical context in which they emerged. It then delves into the technical details of each approach, highlighting their unique characteristics and applications. The author also provides examples of real-world scenarios where one or the other approach may be more appropriate, based on factors such as data availability, computational resources, and desired outcomes. As the book progresses, the author shifts focus to the challenges and limitations of both shallow and deep learning, and how they can be addressed through careful consideration of the underlying assumptions and constraints of each approach. He also offers insights into the future of machine learning, and how it is likely to evolve in response to advances in technology and societal needs. Throughout the book, the author emphasizes the importance of understanding the process of technological evolution, and how this can inform our choices about which approaches to use in different situations. He argues that by studying the history and development of machine learning, we can gain a deeper appreciation for the field's potential and limitations, and make more informed decisions about its application in various domains.
В книге «Shallow arning vs Deep arning A Practical Guide for Machine arning Solutions» рассматриваются различия между поверхностным обучением и глубоким обучением - двумя фундаментальными подходами к машинному обучению, которые произвели революцию в области искусственного интеллекта. Автор, известный эксперт в этой области, дает исчерпывающий обзор этих методов, объясняя их сильные и слабые стороны и предлагая практическое руководство о том, как выбрать правильный подход к вашим конкретным потребностям. Книга начинается с определения ключевых понятий мелкого и глубокого обучения, и обсуждения исторического контекста, в котором они возникли. Затем он углубляется в технические детали каждого подхода, подчеркивая их уникальные характеристики и применения. Автор также приводит примеры реальных сценариев, где тот или иной подход может быть более подходящим, основываясь на таких факторах, как доступность данных, вычислительные ресурсы и желаемые результаты. По мере развития книги автор переключает внимание на проблемы и ограничения как поверхностного, так и глубокого обучения, а также на то, как их можно решить путем тщательного рассмотрения основных предположений и ограничений каждого подхода. Он также предлагает понимание будущего машинного обучения и того, как оно может развиваться в ответ на достижения в области технологий и социальные потребности. На протяжении всей книги автор подчеркивает важность понимания процесса технологической эволюции, и того, как это может информировать наш выбор о том, какие подходы использовать в разных ситуациях. Он утверждает, что, изучая историю и развитие машинного обучения, мы можем глубже оценить потенциал и ограничения этой области и принимать более обоснованные решения о ее применении в различных областях.
Il libro Shallow arning vs Deep arning A Practical Guide for Machine arning Solutions affronta le differenze tra apprendimento superficiale e apprendimento approfondito, due approcci fondamentali per l'apprendimento automatico che hanno rivoluzionato l'intelligenza artificiale. L'autore, un noto esperto in questo campo, fornisce una panoramica completa di questi metodi, spiegando i loro punti di forza e debolezza e offrendo una guida pratica su come scegliere il giusto approccio alle vostre esigenze specifiche. Il libro inizia definendo i concetti chiave dell'apprendimento piccolo e profondo, e discutendo il contesto storico in cui sono nati. Quindi si approfondisce nei dettagli tecnici di ogni approccio, sottolineando le loro caratteristiche e applicazioni uniche. L'autore fornisce anche esempi di scenari reali in cui un approccio può essere più appropriato in base a fattori quali la disponibilità dei dati, le risorse informatiche e i risultati desiderati. Man mano che il libro si sviluppa, l'autore sposta l'attenzione sui problemi e i limiti dell'apprendimento sia superficiale che approfondito, nonché su come risolverli esaminando attentamente i principali presupposti e i limiti di ciascun approccio. Offre anche la comprensione del futuro dell'apprendimento automatico e di come esso possa svilupparsi in risposta ai progressi tecnologici e alle esigenze sociali. Durante tutto il libro, l'autore sottolinea l'importanza di comprendere il processo di evoluzione tecnologica, e il modo in cui può informare le nostre scelte su quali approcci adottare in diverse situazioni. Egli sostiene che, studiando la storia e lo sviluppo dell'apprendimento automatico, possiamo valutare più a fondo il potenziale e i limiti di questo campo e prendere decisioni più fondate su come applicarlo in diversi ambiti.
''
