
BOOKS - Deep Learning at Scale At the Intersection of Hardware, Software, and Data (F...

Deep Learning at Scale At the Intersection of Hardware, Software, and Data (Final Release)
Author: Suneeta Mall
Year: 2024
Format: /RETAIL PDF | EPUB RETAIL COPY
File size: 35.6 MB
Language: ENG
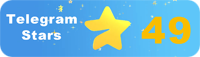
Year: 2024
Format: /RETAIL PDF | EPUB RETAIL COPY
File size: 35.6 MB
Language: ENG
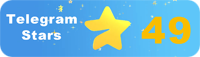
Deep Learning at Scale At the Intersection of Hardware Software and Data Final Release is a book that explores the intersection of hardware, software, and data in deep learning, highlighting the challenges and opportunities that come with scaling up deep learning models to meet the needs of a rapidly changing world. The book provides a comprehensive overview of the current state of deep learning research and its applications in various fields such as computer vision, natural language processing, and speech recognition. It also delves into the technical aspects of deep learning, including the development of new algorithms and architectures, and the use of specialized hardware and software to accelerate training and inference. Additionally, the book discusses the ethical implications of deep learning and its potential impact on society. The book begins by examining the history of deep learning and how it has evolved over time, from its early beginnings to the current state-of-the-art techniques used today. It then delves into the fundamental principles of deep learning, including the different types of neural networks and their applications, and the role of data in driving the success of deep learning models. The book also explores the challenges of scaling up deep learning models, including the need for large amounts of data, computational power, and specialized hardware.
Deep arning at Scale At The Intersection of Hardware Software and Data Final Release - книга, в которой исследуется пересечение аппаратного обеспечения, программного обеспечения и данных в глубоком обучении, освещаются проблемы и возможности, возникающие при масштабировании моделей глубокого обучения для удовлетворения потребностей быстро меняющегося мира. В книге представлен всесторонний обзор текущего состояния исследований в области глубокого обучения и его приложений в различных областях, таких как компьютерное зрение, обработка естественного языка и распознавание речи. Он также углубляется в технические аспекты глубокого обучения, включая разработку новых алгоритмов и архитектур, а также использование специализированного аппаратного и программного обеспечения для ускорения обучения и вывода. Кроме того, в книге обсуждаются этические последствия глубокого обучения и его потенциальное влияние на общество. Книга начинается с изучения истории глубокого обучения и того, как оно развивалось с течением времени, от его ранних истоков до современных современных методов, используемых сегодня. Затем он углубляется в фундаментальные принципы глубокого обучения, включая различные типы нейронных сетей и их приложений, а также роль данных в достижении успеха моделей глубокого обучения. В книге также рассматриваются проблемы масштабирования моделей глубокого обучения, включая потребность в больших объемах данных, вычислительной мощности и специализированном оборудовании.
''
