
BOOKS - Explainable Machine Learning Models and Architectures

Explainable Machine Learning Models and Architectures
Author: Suman Lata Tripathi
Year: September 26, 2023
Format: PDF
File size: PDF 64 MB
Language: English
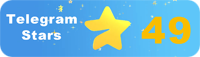
Year: September 26, 2023
Format: PDF
File size: PDF 64 MB
Language: English
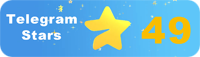
EXPLAINABLE MACHINE LEARNING MODELS AND ARCHITECTURES In today's rapidly evolving technological landscape, it is crucial to understand the development process of modern knowledge and its impact on humanity's survival. The book "Explainable Machine Learning Models and Architectures" provides a comprehensive overview of the cutting-edge advancements in machine learning and deep learning modules, highlighting their significance in various smart and automated systems. As signal processing becomes increasingly reliant on large datasets and complex computations, this text delves into the hardware architecture implementation, software implementation approach, and efficient hardware designs for machine learning applications. The Importance of Efficient Hardware Architectures With the growing demand for realtime applications such as biomedical data analysis in healthcare, smart transportation, satellite image analysis, and IoTenabled systems, there is an urgent need for more powerful yet energyefficient hardware architectures. Traditional hardware architectures often face tradeoffs between power consumption, delay, and IC area, limiting their potential. To overcome these limitations, new hardware accelerators and reconfigurable architectures like graphic processing units (GPUs) and field programmable gate arrays (FPGAs) are being explored. This book offers insights into the development of efficient machine and deep learning models that support highspeed processors with reconfigurable architectures.
ОБЪЯСНИМЫЕ МОДЕЛИ И АРХИТЕКТУРЫ МАШИННОГО ОБУЧЕНИЯ В современном быстро развивающемся технологическом ландшафте крайне важно понимать процесс развития современных знаний и его влияние на выживание человечества. В книге «Explainable Machine arning Models and Architectures» (Объяснимые модели и архитектуры машинного обучения) представлен всесторонний обзор передовых достижений в области машинного обучения и модулей глубокого обучения, подчеркивающий их значимость в различных интеллектуальных и автоматизированных системах. Поскольку обработка сигналов становится все более зависимой от больших наборов данных и сложных вычислений, этот текст углубляется в реализацию аппаратной архитектуры, подход к внедрению программного обеспечения и эффективные аппаратные разработки для приложений машинного обучения. Важность эффективных аппаратных архитектур В связи с растущим спросом на приложения реального времени, такие как анализ биомедицинских данных в здравоохранении, интеллектуальный транспорт, анализ спутниковых изображений и системы IoTenabled, существует острая необходимость в более мощных, но энергоэффективных аппаратных архитектурах. Традиционные аппаратные архитектуры часто сталкиваются с компромиссами между энергопотреблением, задержкой и областью ИС, ограничивая их потенциал. Чтобы преодолеть эти ограничения, изучаются новые аппаратные ускорители и реконфигурируемые архитектуры, такие как графические процессоры (GPU) и программируемые пользователем вентильные матрицы (FPGA). Эта книга предлагает понимание разработки эффективных моделей машинного и глубокого обучения, которые поддерживают высокоскоростные процессоры с реконфигурируемыми архитектурами.
MODÈLES EXPLICITES ET ARCHITECTURES DE L'APPRENTISSAGE AUTOMATIQUE Dans le paysage technologique en évolution rapide d'aujourd'hui, il est essentiel de comprendre le processus de développement des connaissances modernes et leur impact sur la survie de l'humanité. livre « Exploitable Machine arning Models and Architectures » (Modèles et architectures d'apprentissage automatique explicables) présente un aperçu complet des avancées de l'apprentissage automatique et des modules d'apprentissage profond, soulignant leur importance dans divers systèmes intelligents et automatisés. Comme le traitement des signaux est de plus en plus dépendant de grands ensembles de données et de calculs complexes, ce texte s'approfondit dans la mise en œuvre de l'architecture matérielle, l'approche de la mise en œuvre du logiciel et le développement matériel efficace pour les applications d'apprentissage automatique. L'importance D'architectures matérielles efficaces En raison de la demande croissante D'applications en temps réel telles que L'analyse des données biomédicales dans les soins de santé, le transport intelligent, L'analyse des images satellitaires et les systèmes IoTenabled, il est urgent de disposer D'architectures matérielles plus puissantes mais économes en énergie. s architectures matérielles traditionnelles sont souvent confrontées à des compromis entre la consommation d'énergie, la latence et la propriété intellectuelle, limitant leur potentiel. Pour surmonter ces contraintes, on étudie de nouveaux accélérateurs matériels et architectures reconfigurables telles que les GPU et les matrices ventiles programmables par l'utilisateur (FPGA). Ce livre offre une compréhension du développement de modèles d'apprentissage machine et profond efficaces qui prennent en charge les processeurs à haute vitesse avec des architectures reconfigurables.
MODELOS Y ARQUITECTURAS EXPLICABLES DEL APRENDIZAJE AUTOMÁTICO En el panorama tecnológico en rápida evolución actual, es fundamental comprender el proceso de desarrollo del conocimiento moderno y su impacto en la supervivencia de la humanidad. libro «Modelos y Arquitecturas de Aprendizaje de Máquinas Explotables» ofrece una visión general completa de los avances avanzados en el aprendizaje automático y los módulos de aprendizaje profundo, destacando su importancia en varios sistemas inteligentes y automatizados. A medida que el procesamiento de señales se vuelve cada vez más dependiente de grandes conjuntos de datos y computación compleja, este texto profundiza en la implementación de la arquitectura de hardware, el enfoque de implementación de software y el desarrollo de hardware eficiente para aplicaciones de aprendizaje automático. Importancia de las arquitecturas de hardware eficientes Debido a la creciente demanda de aplicaciones en tiempo real, como el análisis de datos biomédicos en salud, el transporte inteligente, el análisis de imágenes satelitales y los sistemas IoTenabled, existe una necesidad urgente de arquitecturas de hardware más potentes pero eficientes en energía. arquitecturas de hardware tradicionales a menudo enfrentan compromisos entre el consumo de energía, la latencia y el área de PI, limitando su potencial. Para superar estas limitaciones, se estudian nuevos aceleradores de hardware y arquitecturas reconfigurables, como las GPU (GPU) y las matrices de válvulas programables por el usuario (FPGA). Este libro ofrece una visión del desarrollo de modelos eficientes de aprendizaje automático y profundo que admiten procesadores de alta velocidad con arquitecturas reconfigurables.
MODELOS EXPLICÁVEIS E ARQUITETURA DE APRENDIZAGEM DE MÁQUINAS Na paisagem tecnológica moderna em rápido desenvolvimento, é fundamental compreender o processo de desenvolvimento do conhecimento moderno e seus efeitos na sobrevivência humana. O livro «Explainable Machine arning Models and Arquitetures» (Modelos Explicáveis e Arquiteturas de Aprendizagem de Máquinas) apresenta uma visão completa dos avanços do aprendizado de máquinas e módulos de aprendizagem profunda que enfatizam sua importância em vários sistemas inteligentes e automatizados. Como o processamento de sinais está cada vez mais dependente de grandes conjuntos de dados e computação complexa, este texto está se aprofundando na implementação da arquitetura de hardware, abordagem de implementação de software e desenvolvimento de hardware eficiente para aplicações de aprendizado de máquina. Com a crescente demanda por aplicações em tempo real, como análise de dados biomédicos na saúde, transporte inteligente, análise de imagens por satélite e sistemas de IoTenabled, a necessidade de arquiteturas de hardware mais potentes, mas eficientes em energia. Arquiteturas tradicionais de hardware muitas vezes enfrentam compromissos entre consumo de energia, atraso e área de PI, limitando seu potencial. Para superar essas limitações, estão sendo estudados novos aceleradores de hardware e arquiteturas reconfiguráveis, como processadores gráficos (GPU) e matrizes de ventela programáveis pelo usuário (FPGA). Este livro oferece compreensão do desenvolvimento de modelos eficientes de aprendizado de máquina e profundidade que suportam processadores de alta velocidade com arquiteturas reconfiguradas.
MODELLI SPIEGABILI E ARCHITETTURE DI APPRENDIMENTO AUTOMATICO In un panorama tecnologico in continua evoluzione, è fondamentale comprendere il processo di sviluppo della conoscenza moderna e il suo impatto sulla sopravvivenza dell'umanità. Il libro «Explainable Machine arning Models and Architectures» fornisce una panoramica completa dei progressi dell'apprendimento automatico e dei moduli di apprendimento approfondito che ne evidenzia l'importanza in diversi sistemi intelligenti e automatizzati. Poiché l'elaborazione dei segnali diventa sempre più dipendente da dataset di grandi dimensioni e da elaborazioni complesse, il testo viene approfondito con l'architettura hardware, l'approccio all'implementazione del software e lo sviluppo hardware efficace per le applicazioni di apprendimento automatico. L'importanza delle architetture hardware efficaci A causa della crescente domanda di applicazioni in tempo reale, come l'analisi dei dati biomedici in ambito sanitario, il trasporto intelligente, l'analisi delle immagini satellitari e i sistemi di IoTenabled, c'è la necessità di architetture hardware più potenti ma efficienti in termini di efficienza energetica. architetture hardware tradizionali spesso affrontano compromessi tra consumo energetico, ritardo e proprietà intellettuale, limitandone il potenziale. Per superare questi limiti, vengono studiati nuovi acceleratori hardware e architetture riconducibili, come i processori grafici (GPU) e le matrici ventile programmabili dall'utente (FPGA). Questo libro offre la comprensione dello sviluppo di modelli di apprendimento automatico e approfondito efficienti che supportano processori ad alta velocità con architetture riconducibili.
ERKLÄRBARE MODELLE UND ARCHITEKTUREN DES MASCHINELLEN LERNENS In der heutigen schnelllebigen technologischen Landschaft ist es von entscheidender Bedeutung, den Entwicklungsprozess des modernen Wissens und seine Auswirkungen auf das Überleben der Menschheit zu verstehen. Das Buch Explainable Machine arning Models and Architectures (Erklärbare Modelle und Architekturen des maschinellen rnens) bietet einen umfassenden Überblick über die neuesten Fortschritte im Bereich des maschinellen rnens und der Deep-arning-Module und unterstreicht deren Bedeutung in verschiedenen intelligenten und automatisierten Systemen. Da die gnalverarbeitung zunehmend von großen Datensätzen und komplexen Berechnungen abhängig wird, vertieft sich dieser Text in die Implementierung einer Hardware-Architektur, einen Ansatz zur Implementierung von Software und effiziente Hardware-Entwicklungen für Machine-arning-Anwendungen. Die Bedeutung effizienter Hardwarearchitekturen Aufgrund der steigenden Nachfrage nach Echtzeitanwendungen wie biomedizinische Datenanalyse im Gesundheitswesen, intelligentem Transport, Satellitenbildanalyse und IoTenabled-Systemen besteht ein dringender Bedarf an leistungsfähigeren, aber energieeffizienteren Hardwarearchitekturen. Herkömmliche Hardwarearchitekturen stoßen häufig auf Kompromisse zwischen Stromverbrauch, Latenz und IC-Bereich, wodurch ihr Potenzial eingeschränkt wird. Um diese Einschränkungen zu überwinden, werden neue Hardwarebeschleuniger und rekonfigurierbare Architekturen wie GPUs (GPUs) und Field Programmable Gate Arrays (FPGAs) untersucht. Dieses Buch bietet Einblicke in die Entwicklung effizienter maschineller und Deep-arning-Modelle, die High-Speed-Prozessoren mit rekonfigurierbaren Architekturen unterstützen.
MACHINE למידת מודלים וארכיטקטורות בנוף הטכנולוגי המתפתח במהירות של ימינו, זה חיוני להבין את התפתחות הידע המודרני ואת השפעתו על הישרדות האדם. הספר Explainable Machine arning Models and Architectures מספק סקירה מקיפה של ההתקדמות האחרונה בלימוד מכונה ומודולי למידה עמוקים, ומדגיש את חשיבותם במערכות אינטליגנטיות ואוטומטיות שונות. כאשר עיבוד אותות נעשה תלוי יותר ויותר במערכות נתונים גדולות וחישובים מורכבים, טקסט זה מתעמק ביישום ארכיטקטורת חומרה, גישה ליישום תוכנה, והתפתחויות חומרה יעילות ליישומי למידת מכונה. חשיבותן של ארכיטקטורות חומרה יעילות עם הביקוש הגובר ליישומים בזמן אמת כמו ניתוח נתונים ביו-רפואיים בתחומי הבריאות, תחבורה אינטליגנטית, ניתוח תמונות לוויין ומערכות IOTENBIED, יש צורך דחוף בארכיטקטורות חומרה חזקות יותר אך יעילות אנרגטית. ארכיטקטורות חומרה מסורתיות מתמודדות לעתים קרובות עם מסחור בין כוח, איחור, ותחום IC, המגביל את הפוטנציאל שלהן. על מנת להתגבר על מגבלות אלו, מאיצי חומרה חדשים וארכיטקטורות שניתן להגדיר מחדש כמו יחידות עיבוד גרפיקה (GPUs) ומערכי שער ניתנים לתכנות שדה (FPGAs) נחקרים. הספר מציע תובנות על פיתוח מכונה יעילה ומודלים ללמידה עמוקה התומכים במעבדים במהירות גבוהה עם ארכיטקטורה ניתנת לשינוי.''
AÇIKLANABİLİR MACHINE LEARNING MODELS AND ARCHITECTURES Günümüzün hızla gelişen teknolojik ortamında, modern bilginin gelişimini ve insan yaşamı üzerindeki etkisini anlamak çok önemlidir. "Açıklanabilir Makine Öğrenme Modelleri ve Mimarileri" kitabı, makine öğrenimi ve derin öğrenme modüllerindeki en son gelişmelere kapsamlı bir genel bakış sunarak, çeşitli akıllı ve otomatik sistemlerdeki önemini vurgulamaktadır. nyal işleme büyük veri kümelerine ve karmaşık hesaplamalara giderek daha fazla bağımlı hale geldikçe, bu metin donanım mimarisi uygulamasına, yazılım uygulama yaklaşımına ve makine öğrenimi uygulamaları için verimli donanım geliştirmelerine odaklanmaktadır. Verimli donanım mimarilerinin önemi Sağlık hizmetlerinde biyomedikal veri analizi, akıllı ulaşım, uydu görüntü analizi ve IoTenabled sistemleri gibi gerçek zamanlı uygulamalara olan talebin artmasıyla birlikte, daha güçlü ancak enerji verimli donanım mimarilerine acil bir ihtiyaç vardır. Geleneksel donanım mimarileri genellikle güç, gecikme ve IC etki alanı arasındaki takaslarla karşı karşıya kalır ve potansiyellerini sınırlar. Bu sınırlamaların üstesinden gelmek için, yeni donanım hızlandırıcıları ve grafik işleme birimleri (GPU'lar) ve alan programlanabilir kapı dizileri (FPGA'lar) gibi yeniden yapılandırılabilir mimariler araştırılmaktadır. Bu kitap, yeniden yapılandırılabilir mimarilere sahip yüksek hızlı işlemcileri destekleyen verimli makine ve derin öğrenme modellerinin geliştirilmesine ilişkin bilgiler sunmaktadır.
نماذج ومهندسات التعلم الآلي القابلة للتفسير في المشهد التكنولوجي سريع التطور اليوم، من الأهمية بمكان فهم تطور المعرفة الحديثة وتأثيرها على بقاء الإنسان. يقدم كتاب «نماذج ومعماريات التعلم الآلي القابلة للتفسير» نظرة عامة شاملة على أحدث التطورات في التعلم الآلي ووحدات التعلم العميق، مما يسلط الضوء على أهميتها في مختلف الأنظمة الذكية والآلية. نظرًا لأن معالجة الإشارات أصبحت تعتمد بشكل متزايد على مجموعات البيانات الكبيرة والحسابات المعقدة، فإن هذا النص يتعمق في تنفيذ بنية الأجهزة ونهج تنفيذ البرامج والتطورات الفعالة للأجهزة لتطبيقات التعلم الآلي. أهمية بنية الأجهزة الفعالة مع الطلب المتزايد على التطبيقات في الوقت الفعلي مثل تحليل البيانات الطبية الحيوية في الرعاية الصحية والنقل الذكي وتحليل صور الأقمار الصناعية وأنظمة IoTenabled، هناك حاجة ملحة لهياكل أجهزة أكثر قوة ولكنها موفرة للطاقة. غالبًا ما تواجه بنى الأجهزة التقليدية مقايضات بين الطاقة والكمون ومجال IC، مما يحد من إمكاناتها. للتغلب على هذه القيود، يتم استكشاف مسرعات الأجهزة الجديدة والبنى القابلة لإعادة التشكيل مثل وحدات معالجة الرسومات (GPUs) ومصفوفات البوابات القابلة للبرمجة الميدانية (FPGAs). يقدم هذا الكتاب رؤى حول تطوير نماذج فعالة للتعلم الآلي والعميق تدعم المعالجات عالية السرعة ذات الهياكل القابلة لإعادة التشكيل.
EXPLAINABLE MACHINE LEARNING MODELS and ARCHITECTURES 오늘날의 빠르게 진화하는 기술 환경에서 현대 지식의 발전과 인간 생존에 미치는 영향을 이해하는 것이 중요합니다. "설명 가능한 머신 러닝 모델 및 아키텍처" 책은 머신 러닝 및 딥 러닝 모듈의 최신 발전에 대한 포괄적 인 개요를 제공하여 다양한 지능형 및 자동화 시스템에서의 중요성을 강조합니다. 신호 처리가 대규모 데이터 세트 및 복잡한 계산에 점점 더 의존함에 따라이 텍스트는 하드웨어 아키텍처 구현, 소프트웨어 구현 접근 방식 및 머신 러닝 응용 프로그램을위한 효율적인 하드웨어 효율적인 하드웨어 아키텍처의 중요성 의료, 지능형 운송, 위성 이미지 분석 및 IoTabiled 시스템의 생의학 데이터 분석과 같은 실시간 응용 분야에 대한 수요가 증가함에 따라보다 강력하지만 에너지 효율적인 하드웨어 아키텍처가 절실히 필요합니다. 기존의 하드웨어 아키텍처는 종종 전력, 대기 시간 및 IC 도메인 간의 상충 관계에 직면하여 잠재력을 제한합니다. 이러한 한계를 극복하기 위해 그래픽 처리 장치 (GPU) 및 필드 프로그래밍 가능 게이트 어레이 (FPGA) 와 같은 새로운 하드웨어 가속기 및 재구성 가능한 아키텍처를 탐색하고 있습니다. 이 책은 재구성 가능한 아키텍처로 고속 프로세서를 지원하는 효율적인 머신 및 딥 러닝 모델 개발에 대한 통찰력을 제공합니다.
在當今快速發展的技術環境中,了解現代知識的發展過程及其對人類生存的影響至關重要。「可解釋的機器學習模型和體系結構」一書全面概述了機器學習和深度學習模塊方面的先進進展,強調了它們在各種智能和自動化系統中的重要性。隨著信號處理越來越依賴於大型數據集和復雜的計算,本文深入研究了硬件體系結構的實現,軟件實施方法和機器學習應用程序的有效硬件開發。高效硬件體系結構的重要性隨著對實時應用程序的需求不斷增長,例如醫療保健中的生物醫學數據分析、智能傳輸、衛星圖像分析和IoTabled系統,迫切需要更強大但節能的硬件體系結構。傳統的硬件體系結構通常在功耗,延遲和IC區域之間面臨權衡,從而限制了其潛力。為了克服這些限制,正在研究新的硬件加速器和可重新配置的體系結構,例如GPU(GPU)和用戶可編程通風矩陣(FPGA)。本書提供了開發高效機器和深度學習模型的見解,該模型支持具有可重新配置體系結構的高速處理器。
