
BOOKS - Split Federated Learning for Secure IoT Applications Concepts, frameworks, ap...

Split Federated Learning for Secure IoT Applications Concepts, frameworks, applications and case studies
Author: Gururaj Harinahalli Lokesh, Geetabai S. Hukkeri, N.Z. Jhanjhi, Hong Lin
Year: 2024
Format: PDF
File size: 10.1 MB
Language: ENG
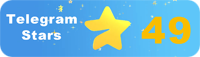
Year: 2024
Format: PDF
File size: 10.1 MB
Language: ENG
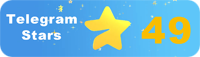
The book "Split Federated Learning for Secure IoT Applications Concepts, Frameworks, Applications, and Case Studies" provides a comprehensive overview of the current state of federated learning research and its applications in secure IoT applications. The book covers the fundamental concepts, frameworks, and applications of split federated learning, as well as case studies that demonstrate the practicality and effectiveness of this approach. It emphasizes the importance of understanding the technological process of developing modern knowledge as the basis for the survival of humanity and the unity of people in a warring state. The book begins by discussing the challenges of traditional federated learning and how split federated learning addresses these challenges. It then delves into the basic concepts of split federated learning, including data heterogeneity, communication overhead, and privacy concerns. The authors explain how split federated learning can be used to improve the security and privacy of IoT applications, such as edge computing, smart cities, and industrial control systems. They also highlight the potential benefits of using split federated learning in these applications, such as improved accuracy, reduced communication overhead, and enhanced privacy.
В книге «Split Federated arning for Secure IoT Applications Concepts, Framework, Applications, and Case Studies» (Разделение федеративного обучения для безопасных приложений Интернета вещей: концепции, платформы, приложения и примеры внедрения) представлен всесторонний обзор текущего состояния исследований федеративного обучения и его приложений в безопасных приложениях Интернета вещей. Книга охватывает фундаментальные концепции, основы и приложения разделенного федеративного обучения, а также тематические исследования, которые демонстрируют практичность и эффективность этого подхода. В нем подчеркивается важность понимания технологического процесса развития современных знаний как основы выживания человечества и единства людей в воюющем государстве. Книга начинается с обсуждения проблем традиционного федеративного обучения и того, как разделенное федеративное обучение решает эти проблемы. Затем он углубляется в основные концепции разделенного федеративного обучения, включая неоднородность данных, накладные расходы на связь и проблемы конфиденциальности. Авторы объясняют, как разделенное федеративное обучение может быть использовано для повышения безопасности и конфиденциальности приложений интернета вещей, таких как периферийные вычисления, умные города и промышленные системы управления. Они также подчеркивают потенциальные преимущества использования раздельного объединенного обучения в этих приложениях, такие как повышенная точность, снижение затрат на связь и повышение конфиденциальности.
''
